DREAMS: deep read-level error model for sequencing data applied to low-frequency variant calling and circulating tumor DNA detection, Genome Biology
Por um escritor misterioso
Last updated 03 junho 2024
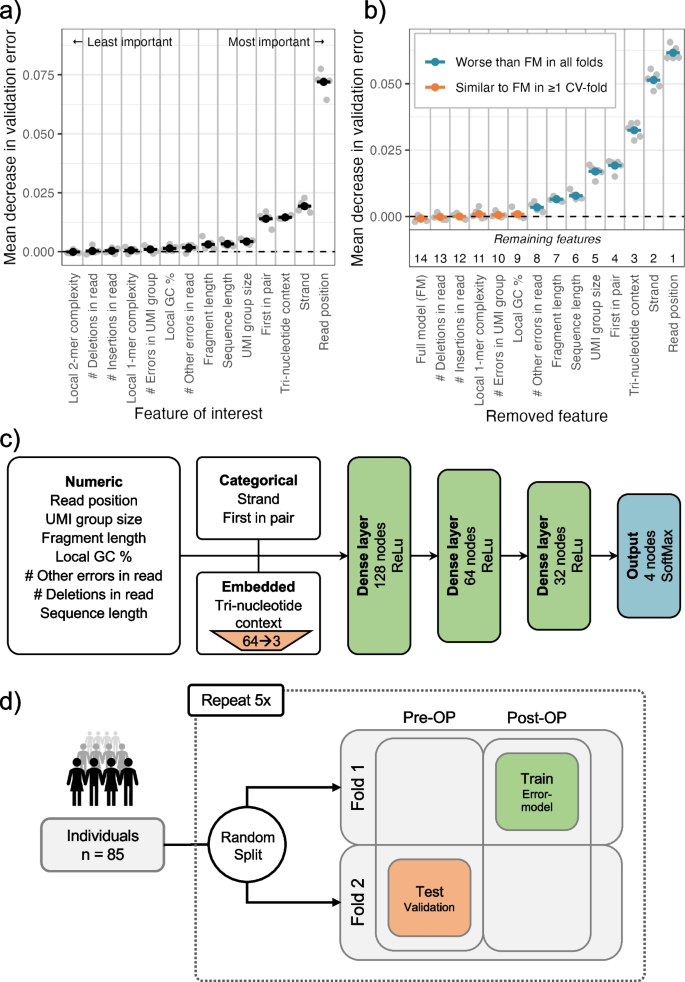
Circulating tumor DNA detection using next-generation sequencing (NGS) data of plasma DNA is promising for cancer identification and characterization. However, the tumor signal in the blood is often low and difficult to distinguish from errors. We present DREAMS (Deep Read-level Modelling of Sequencing-errors) for estimating error rates of individual read positions. Using DREAMS, we develop statistical methods for variant calling (DREAMS-vc) and cancer detection (DREAMS-cc). For evaluation, we generate deep targeted NGS data of matching tumor and plasma DNA from 85 colorectal cancer patients. The DREAMS approach performs better than state-of-the-art methods for variant calling and cancer detection.
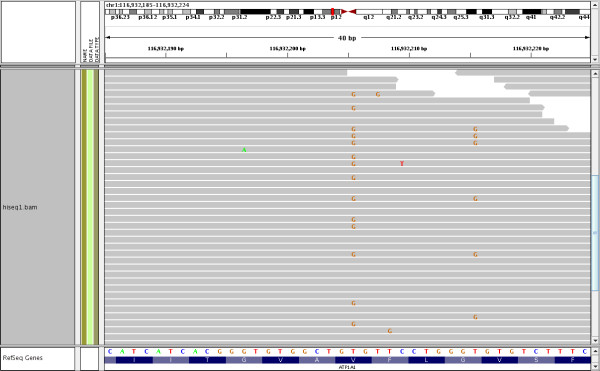
Systematic errors in HiSeq data. A screenshot from the IGV browser
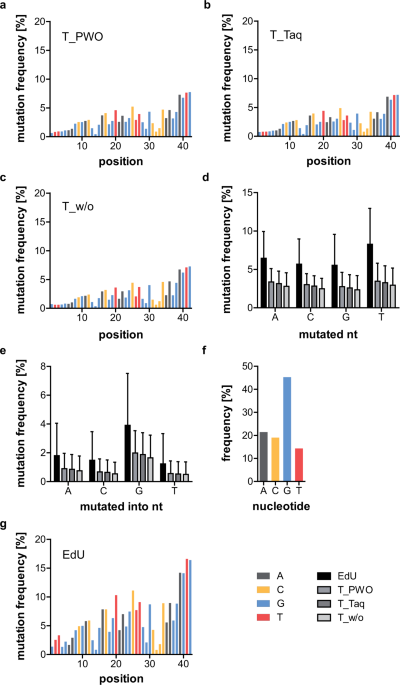
Systematic evaluation of error rates and causes in short samples

Integration of intra-sample contextual error modeling for improved
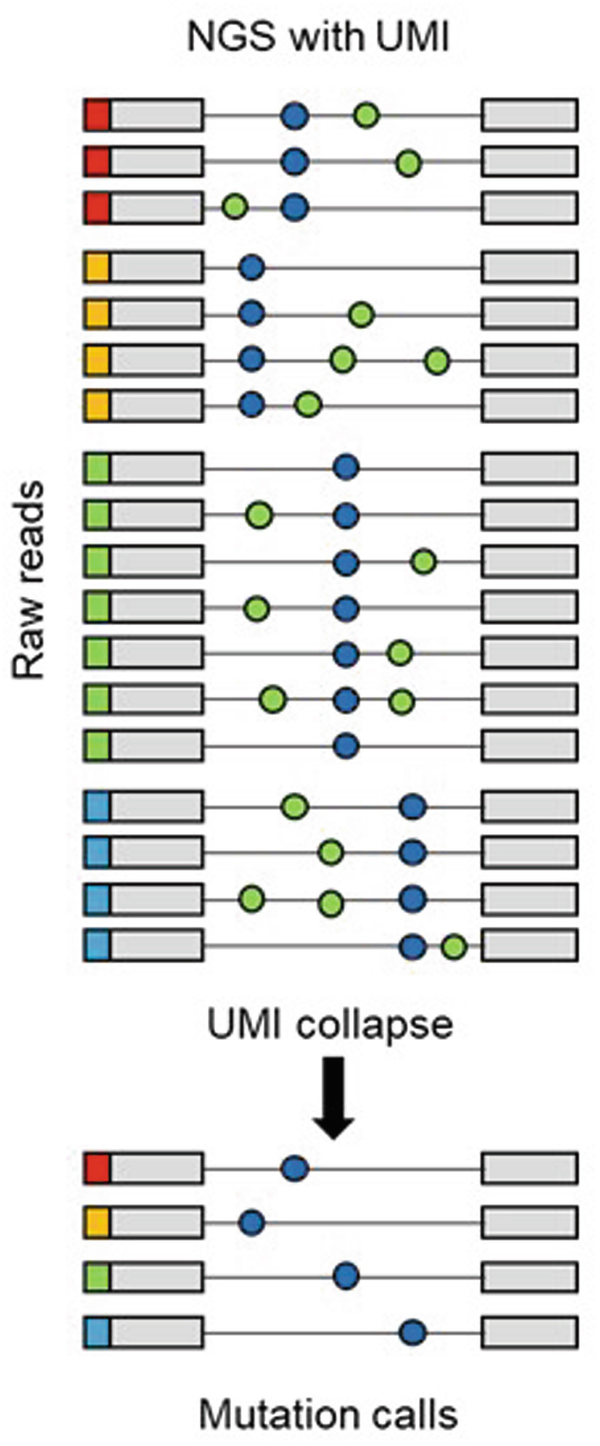
NGS-Based Tumor-Informed Analysis of Circulating Tumor DNA

DREAMS: Deep Read-level Error Model for Sequencing data applied to

LFMD: detecting low-frequency mutations in high-depth genome
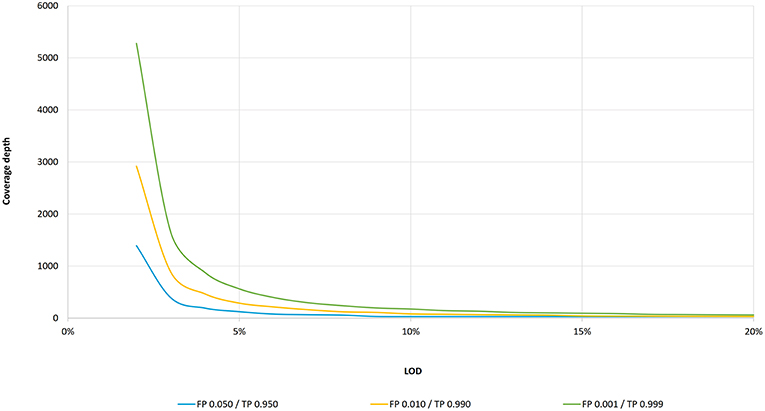
Frontiers Standardization of Sequencing Coverage Depth in NGS
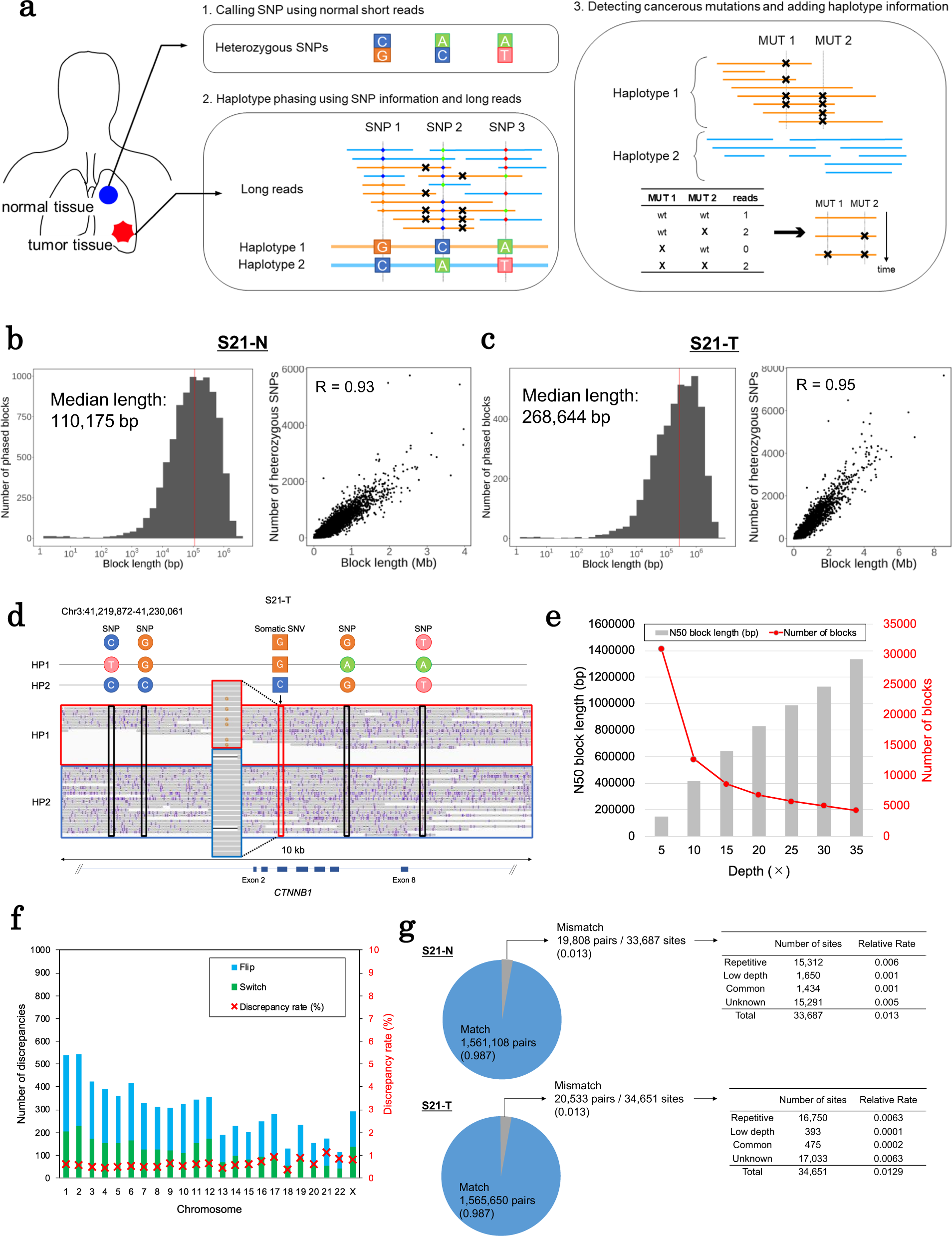
Phasing analysis of lung cancer genomes using a long read

Detecting and Quantitating Low Fraction DNA Variants with Low
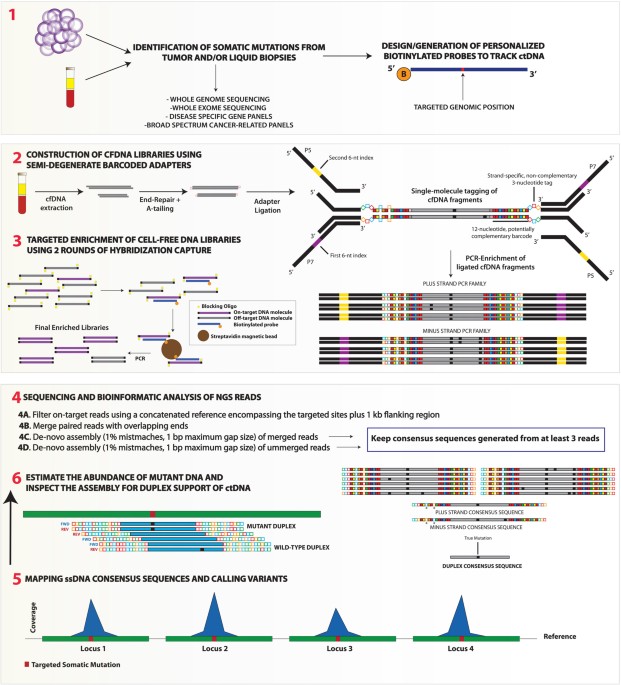
Targeted error-suppressed quantification of circulating tumor DNA
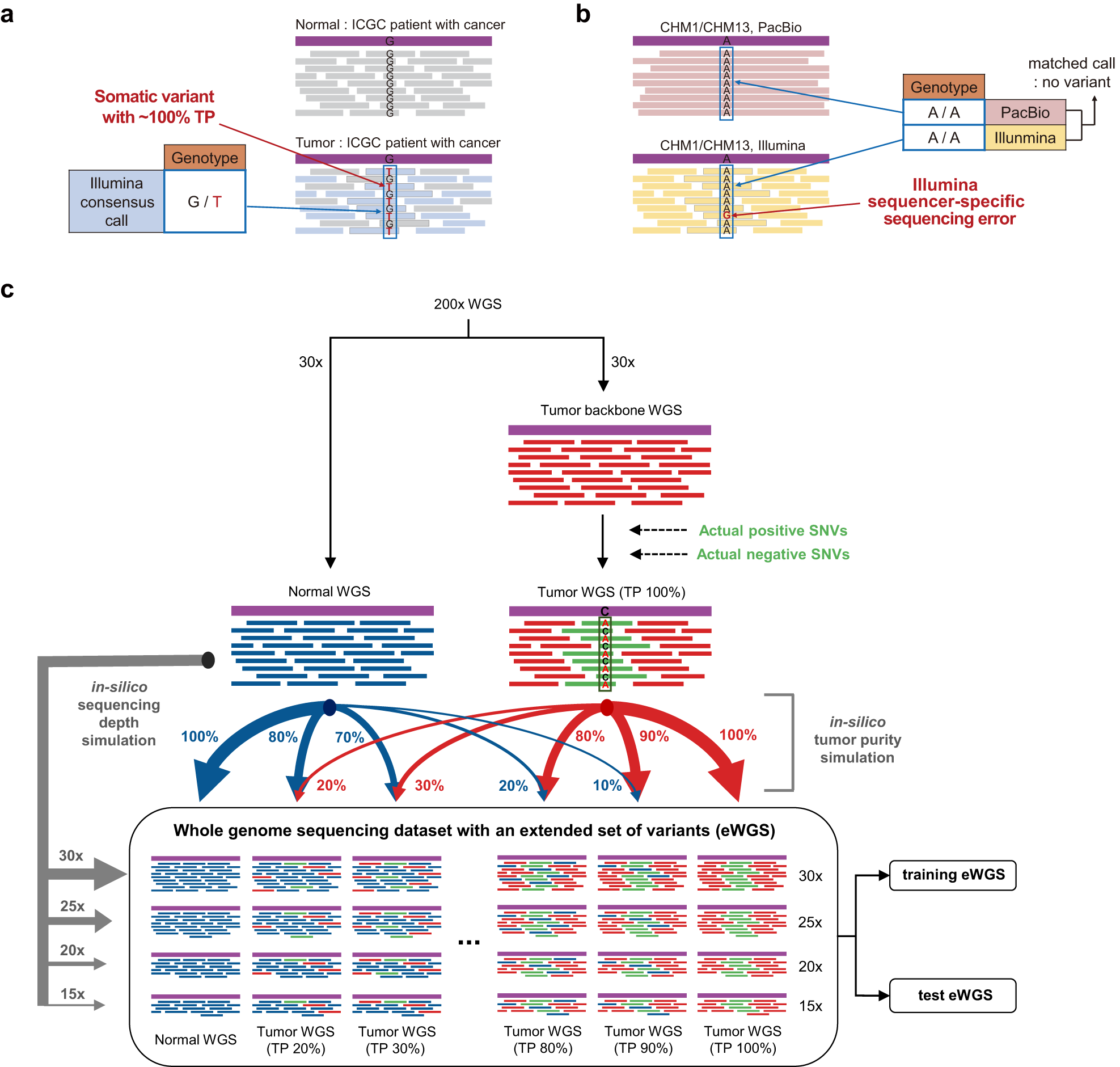
AIVariant: a deep learning-based somatic variant detector for

Accurate detection of circulating tumor DNA using nanopore
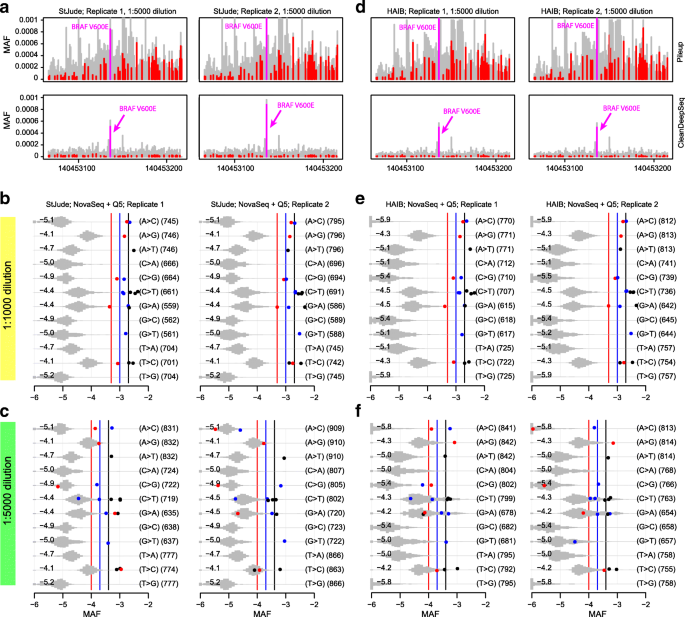
Analysis of error profiles in deep next-generation sequencing data
Recomendado para você
-
SCP-7143-J - The Doorknob (TikTok Variant)03 junho 2024
-
SCP-7143 – J has breached containment : r/DankMemesFromSite1903 junho 2024
-
scp #scpshowerthoughts #minecraft #minecraftparkour #scp173 #scpsecre03 junho 2024
-
Why my pp hard : r/DankMemesFromSite1903 junho 2024
-
S I M P SCP Foundation (RP) Amino03 junho 2024
-
Pride month logo, SCP Foundation03 junho 2024
-
watched rick and morty thought of this SCP - Imgflip03 junho 2024
-
Miscellaneous SCPs, SCP Meta Wiki03 junho 2024
-
Journal of Comparative Neurology, Systems Neuroscience Journal03 junho 2024
-
Solubility Enhancement of Hydrophobic Substances in Water/Cyrene Mixtures: A Computational Study03 junho 2024
você pode gostar
-
Desapego Games - Steam > CONTA STEAM / 4.8 ANOS / 132 JOGOS / COM 1 BANIMENTO NO CS GO FREE03 junho 2024
-
Ver Os Cavaleiros do Zodíaco (HD) Dublado Online Grátis Completo03 junho 2024
-
One piece Episode 1026 Fight Scene03 junho 2024
-
Lounge review, 'Assassin's Creed: Valhalla' is a 100-hour game worth your time and money03 junho 2024
-
Aoashi Anime Boys GIF - Aoashi Anime Boys Hot Anime Boys - Discover & Share GIFs03 junho 2024
-
Soul Origin03 junho 2024
-
PC Longplay [078] Prince of Persia: The Sands of Time (Part 1 of 503 junho 2024
-
Quantos episódios tem a 5ª temporada de Pokémon? – Respondedor03 junho 2024
-
Chainsaw Man -『Dogland』10° Encerramento COMPLETO PT/BR03 junho 2024
-
Boneca Barbie O Filme Barbieland Mattel - Boneca Barbie - Magazine03 junho 2024